Error analysis of the global pre-processing workflow
Contents
Error analysis of the global pre-processing workflow#
Here we reproduce the error analysis shown in Maussion et al. (2019) for the pre-processing part only, and for the glacier directories (version 1.4). The error analysis of user runs needs a separate handling, see the deal_with_errors notebook for more information.
Get the files#
We download the glacier_statistics
files from the preprocessed directories folders, at the level 5. That is, we are going to count all errors that happened during the pre-processing chain.
from oggm import utils
import pandas as pd
import seaborn as sns
# This is CRU + centerlines. But you can try CRU+elev_bands, or ERA5+elev_bands, etc!
url = 'https://cluster.klima.uni-bremen.de/~oggm/gdirs/oggm_v1.4/L3-L5_files/CRU/centerlines/qc3/pcp2.5/no_match/RGI62/b_040/L5/summary/'
# this can take some time
df = []
for rgi_reg in range(1, 19):
fpath = utils.file_downloader(url + f'glacier_statistics_{rgi_reg:02d}.csv')
df.append(pd.read_csv(fpath, index_col=0, low_memory=False))
df = pd.concat(df, sort=False).sort_index()
Analyze the errors#
sns.countplot(y="error_task", data=df);
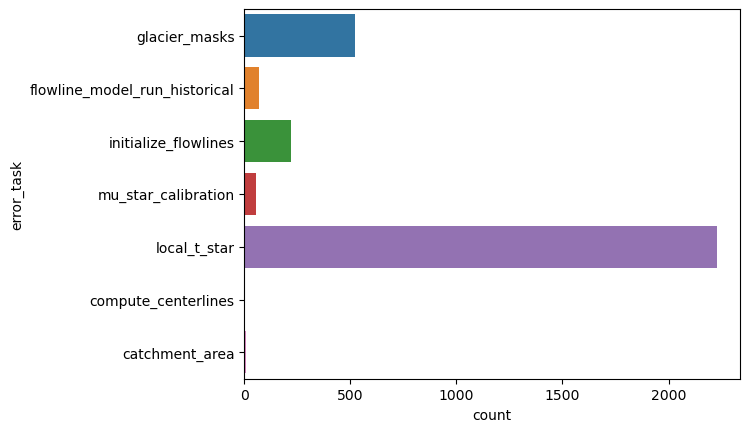
"% area errors all sources: {:.2f}%".format(df.loc[~df['error_task'].isnull()].rgi_area_km2.sum() / df.rgi_area_km2.sum() * 100)
'% area errors all sources: 3.24%'
Most errors occur because the interpolation of tstar did not work.
We now look at the errors that occur already before applying the climate tasks:
dfe = df.loc[~df['error_task'].isnull()]
dfe = dfe.loc[~dfe['error_task'].isin(['local_t_star', 'mu_star_calibration'])]
"% area errors before climate: {:.2f}%".format(dfe.rgi_area_km2.sum() / df.rgi_area_km2.sum() * 100)
'% area errors before climate: 0.41%'
sns.countplot(y="error_task", data=dfe);
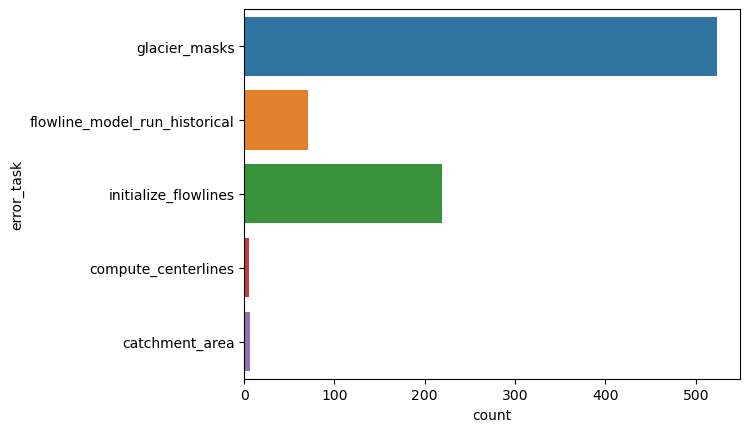
# 15 largest glaciers
df.loc[~df['error_task'].isnull()].sort_values(by='rgi_area_km2', ascending=False)[['rgi_area_km2', 'error_task', 'error_msg']].iloc[:15]
rgi_area_km2 | error_task | error_msg | |
---|---|---|---|
rgi_id | |||
RGI60-17.15804 | 790.282 | mu_star_calibration | MassBalanceCalibrationError: RGI60-17.15804 mu... |
RGI60-17.15808 | 721.950 | local_t_star | MassBalanceCalibrationError: RGI60-17.15808: m... |
RGI60-13.54431 | 663.729 | flowline_model_run_historical | RuntimeError: CFL error: required time step sm... |
RGI60-17.15897 | 428.505 | local_t_star | MassBalanceCalibrationError: RGI60-17.15897: m... |
RGI60-05.10735 | 392.607 | local_t_star | MassBalanceCalibrationError: RGI60-05.10735: m... |
RGI60-05.10634 | 388.067 | local_t_star | MassBalanceCalibrationError: RGI60-05.10634: m... |
RGI60-05.10617 | 373.290 | local_t_star | MassBalanceCalibrationError: RGI60-05.10617: m... |
RGI60-05.10762 | 309.061 | local_t_star | MassBalanceCalibrationError: RGI60-05.10762: m... |
RGI60-05.10748 | 289.188 | local_t_star | MassBalanceCalibrationError: RGI60-05.10748: m... |
RGI60-13.43483 | 282.721 | flowline_model_run_historical | RuntimeError: CFL error: required time step sm... |
RGI60-17.15898 | 268.749 | local_t_star | MassBalanceCalibrationError: RGI60-17.15898: m... |
RGI60-01.16377 | 254.841 | flowline_model_run_historical | RuntimeError: CFL error: required time step sm... |
RGI60-05.10988 | 207.766 | local_t_star | MassBalanceCalibrationError: RGI60-05.10988: m... |
RGI60-14.04404 | 203.336 | flowline_model_run_historical | RuntimeError: CFL error: required time step sm... |
RGI60-05.10959 | 183.850 | local_t_star | MassBalanceCalibrationError: RGI60-05.10959: m... |
Example error comparison#
CRU vs ERA5:
# ERA5 uses a different precipitation factor
url = 'https://cluster.klima.uni-bremen.de/~oggm/gdirs/oggm_v1.4/L3-L5_files/ERA5/centerlines/qc3/pcp1.6/no_match/RGI62/b_040/L5/summary/'
# this can take some time
df_era5 = []
# we don't look at RGI19 (Antarctic glaciers), because no climate available for CRU
for rgi_reg in range(1, 19):
fpath = utils.file_downloader(url + f'glacier_statistics_{rgi_reg:02d}.csv')
df_era5.append(pd.read_csv(fpath, index_col=0, low_memory=False))
df_era5 = pd.concat(df_era5, sort=False).sort_index()
"% area errors all sources for ERA5 is {:.2f}% compared to CRU ({:.2f}%)".format(df_era5.loc[~df_era5['error_task'].isnull()].rgi_area_km2.sum() / df_era5.rgi_area_km2.sum() * 100,
df.loc[~df['error_task'].isnull()].rgi_area_km2.sum() / df.rgi_area_km2.sum() * 100)
'% area errors all sources for ERA5 is 0.92% compared to CRU (3.24%)'
more than three times less errors from the climate tasks occur when using ERA5 than when using CRU !
centerlines vs elevation bands:
# CRU elevation bands
url = 'https://cluster.klima.uni-bremen.de/~oggm/gdirs/oggm_v1.4/L3-L5_files/CRU/elev_bands/qc3/pcp2.5/no_match/RGI62/b_040/L5/summary/'
# this can take some time
df_elev = []
# we don't look at RGI19 (Antarctic glaciers), because no climate available for CRU
for rgi_reg in range(1, 19):
fpath = utils.file_downloader(url + f'glacier_statistics_{rgi_reg:02d}.csv')
df_elev.append(pd.read_csv(fpath, index_col=0, low_memory=False))
df_elev = pd.concat(df_elev, sort=False).sort_index()
"% area errors all sources for elevation band flowlines is {:.2f}% compared to centerlines ({:.2f}%) with CRU".format(df_elev.loc[~df_elev['error_task'].isnull()].rgi_area_km2.sum() / df_elev.rgi_area_km2.sum() * 100,
df.loc[~df['error_task'].isnull()].rgi_area_km2.sum() / df.rgi_area_km2.sum() * 100)
'% area errors all sources for elevation band flowlines is 2.46% compared to centerlines (3.24%) with CRU'
less errors occur when using elevation band flowlines than when using centerlines !
What’s next?#
A more detailed analysis about the type, amount and relative failing glacier area (in total and per RGI region) can be found in this error analysis jupyter notebook. It also includes an error analysis for different MB calibration and climate quality check methods.
If you are interested in how the “common” non-failing glaciers differ in terms of historical volume change, total mass change and specific mass balance between different pre-processed glacier directories, you can check out this jupyter notebook.
return to the OGGM documentation
back to the table of contents